Breaking New Ground: SiMa.ai's Unprecedented Advances in MLPerf™ Benchmarks
Key Takeaways
- Since SiMa’s prior submission to MLPerf Benchmarks in April 2023, we achieved a 20% improvement in our MLPerf Closed Edge Power. (ratio of 3.1-0131 to 3.0-0104 SingleStream on the power metric[1]). In addition, in this latest round we demonstrated up to 85% greater efficiency compared to our leading competitors (ratio of 3.1-0131 to 3.1-0115 on the MultiStream power metric[2]).
- SiMa’s ability to achieve unparalleled power efficiency without compromising performance is a result of its custom-made ML Accelerator and we’re proud to pass along these performance enhancements to offer our customers more value and versatility across a wide range of applications.
- SiMa.ai is committed to consistent participation and performance in MLPerf, where we will transition to more advanced technologies in future generations and continue pushing the boundaries of what’s possible in edge AI.
At SiMa, we focus our efforts on being at the forefront of edge AI technology, consistently pushing the boundaries of both performance and energy efficiency. In today’s rapidly evolving tech landscape, benchmarks serve as critical indicators of a product’s capabilities and competitiveness. That’s why we’re particularly excited to share a comprehensive technical analysis of our most recent MLPerf™ results. These results are groundbreaking: we’ve achieved a 20% improvement in our MLPerf Closed Edge Power score since our last submission in April 2023 (ratio of 3.1-0131 to 3.0-0104 SingleStream on the power metric[1]) and have demonstrated up to 85% greater efficiency compared to our leading competitors (ratio of 3.1-0131 to 3.1-0115 on the MultiStream power metric[2]). This post aims to provide an in-depth look at the metrics, our technologies and the strategies fueling these improvements. We will also show how our performance compares to key industry players.
In the edge ML segment, where both performance and energy efficiency are paramount, our standout metric is FPS/Watt. This metric measures how many frames our system can process per watt of electricity consumed. This is critical for edge AI workloads, where energy constraints can be fixed and pose significant challenges. Our purpose built ML Accelerator is the linchpin of our success in achieving unparalleled power efficiency. Engineered for simplicity, this accelerator is statically scheduled for high utilization, resulting in low power consumption without compromising performance. It’s not just about doing more; it’s about doing more with less—less energy, less cost, and less environmental impact. This focus on power efficiency not only sets us apart from the competition; it positions us as a market leader in a world increasingly concerned with sustainable, efficient computing.
When it comes to performance evaluation, benchmarks are indispensable, and MLPerf is the gold standard for assessing machine learning capabilities, with strict guidelines and a peer based compliance review SiMa.ai participated in the edge inference, closed power division of this rigorous benchmarking process, focusing specifically on the image classification benchmark Resnet50. The choice of Resnet50 is widely recognized as the most widely tested MLbenchmark, providing a comprehensive measure of a system’s machine learning performance for computer vision use cases. To offer a holistic view of our capabilities, we didn’t stop at measuring Frames Per Second (FPS). We also meticulously recorded power consumption metrics across various real-world scenarios, including single-camera setups (“SingleStream”), multi-camera configurations (“Batch 8 or MultiStream”), and data center workloads (“Batch 24 or Offline”). This multifaceted approach ensures that our performance metrics are genuinely indicative of how our technology performs under diverse and demanding loading conditions.
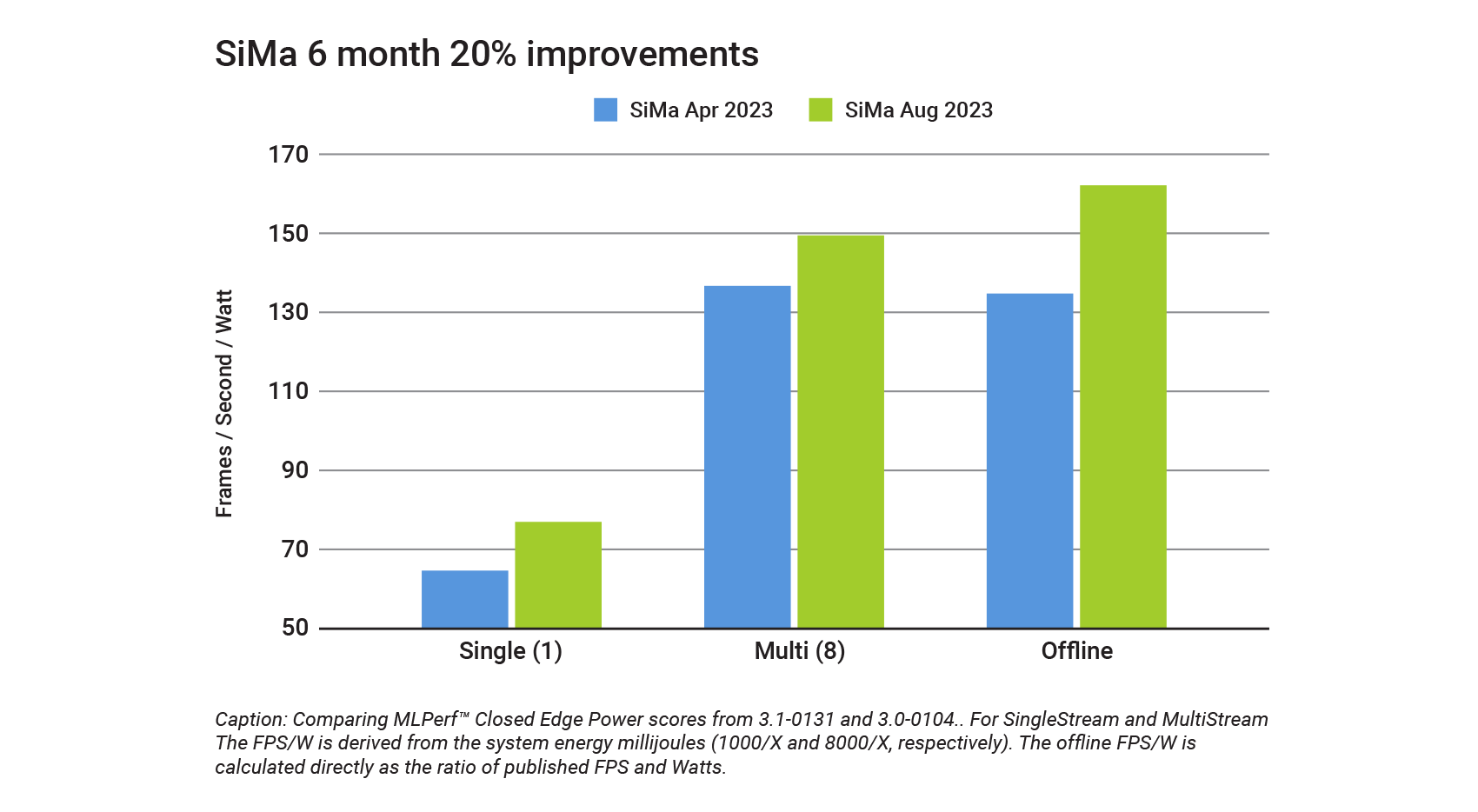
One of the most striking aspects of our latest MLPerf results is the notable 20% improvement in our score since our April 2023 submission [1]. This isn’t a minor tweak or a marginal gain; it’s a significant leap that underscores our commitment to continuous innovation. The driving forces behind this improvement are multifaceted but can be primarily attributed to substantial enhancements in our compiler technology and memory management systems. These foundational improvements optimize code execution and resource allocation, boosting the overall performance of our hardware for any type ML network, not just ResNet50. This is exciting in that these improvements are not benchmark-specific; they translate to real-world benefits, enhancing the performance of all models compiled for our hardware, offering our customers more value and versatility across a wide range of applications.
For those interested in the technical aspects driving our recent performance gains, the architecture of our ML purpose-built Machine Learning Accelerator, plays a pivotal role in supporting the ability to produce an efficient compiler. Optimal performance hinges on smartly allocating operations and data movement within various chip components. Our production compiler takes on this task automatically. Recent enhancements to the compiler have led to more intelligent decision-making in operational allocation, contributing to our improved MLPerf scores. Specifically, we’ve minimized the overhead in our on-chip runtime environment, optimized the compiler to emit more efficient implementations of the argmax function, and streamlined data transfers by reducing memory copy times and eliminating superfluous data movements. These compiler improvements drove a 34.8% improvement to FPS, elevating our Offline FPS from 2,190.27 in version 3.0 to 2,952.58 in version 3.1 [3].
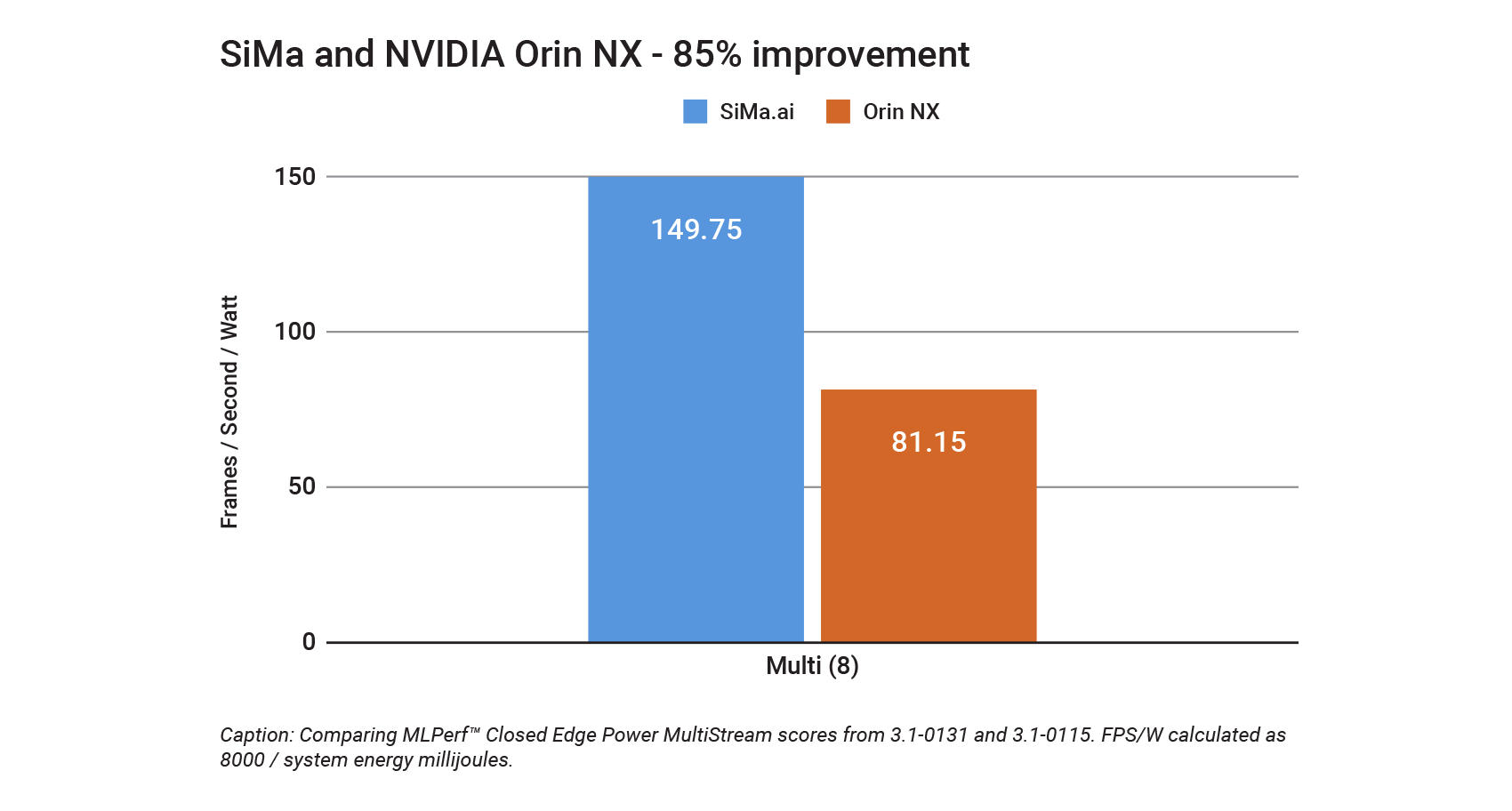
In the fiercely competitive world of edge AI, standing out against industry giants is no small feat. That’s why our latest MLPerf results are particularly noteworthy: they show an astonishing 85% lead against NVIDIA’s newest Jetson NX [2]. This is not just a marginal win; it’s a resounding victory that significantly positions SiMa.ai as a dominant player in the competitive landscape. This 85% lead is monumental for several reasons. First, it underscores the effectiveness of our technology in delivering unparalleled performance and efficiency, even when compared to products from well-established competitors like NVIDIA. Second, it serves as a compelling selling point for potential customers evaluating various edge AI solutions. They can now make data-driven decisions based on these benchmark results, confident that they are opting for a solution that has proven its mettle. In a market where performance and efficiency are paramount, this substantial lead translates into being able to ship your product in that small form factor or not, it can mean a lower total cost of ownership as you scale production; it’s not a declaration of our technological superiority but a promise of the value we bring to our customers.
SiMa.ai is not a flash in the pan in the realm of MLPerf benchmarks; we are a recurring player with a long-term vision. Our consistent participation and performance in these benchmarks are part of a broader growth strategy that extends far beyond just one or two product cycles. Specifically, we are in the process of transitioning from the MLSoC’s 16nm process to a roadmap of products with more advanced technologies in future generations. This move is not merely a technical upgrade; it’s a strategic evolution aimed at ensuring that we continue to lead in performance, efficiency, and innovation. Our commitment to continuous improvement is unwavering and backed by substantial investments in research and development. We understand that in the fast-paced world of edge ML, resting on one’s laurels is not an option. That’s why we are relentlessly focused on pushing the boundaries of what’s possible, ensuring that we remain at the forefront of technological advancements for years to come.
In conclusion, our latest MLPerf results are a powerful testament to SiMa.ai’s unwavering commitment to excellence in both performance and power efficiency. We’ve demonstrated a significant 20% improvement in our score since April 2023, achieved an 85% lead against NVIDIA’s newest Jetson NX, and underscored our unique value proposition with our FPS/Watt metric. These accomplishments are part of a consistent pattern of innovation and growth. Our long-term strategy, which includes transitioning to more advanced technologies, ensures that we are not just keeping pace with the industry but setting the pace. These results collectively affirm our position as a leader in the edge ML space and validate our commitment to delivering solutions that are not just cutting-edge but also sustainable and efficient. As we look to the future, our focus remains clear: to continue pushing the boundaries of what’s possible in edge AI technology.
We invite you to explore our detailed MLPerf results for a deeper understanding of our capabilities. For partnerships or more information, feel free to reach out to us. Also, consider following SiMa.ai for future updates on our technology and achievements.Footnotes:
[1] Verified MLPerf™ score of v3.1 Inference Closed Power ResNet50. [TODO: Webpage link] [TODO Result Row ID] The MLPerf™ name and logo are trademarks of MLCommons Association in the United States and other countries. All rights reserved. Unauthorized use strictly prohibited. See www.mlcommons.org for more information.
[2] Verified MLPerf™ score of v3.1 Inference Closed Power ResNet50. [TODO: Webpage link] [TODO Result Row ID] The MLPerf™ name and logo are trademarks of MLCommons Association in the United States and other countries. All rights reserved. Unauthorized use strictly prohibited. See www.mlcommons.org for more information.
[3] Comparing Offline frames per second as measured from results 3.1-0131 and 3.0-0104. Computed as 2952.58 / 2190.27.